Acoustic tracking is widely used by marine biologists to study the distributions and movements of fishes and marine mammals on time scales ranging from hours to months or even years. Highly powerful, the technique allows scientists to map the migration routes of salmon, or correlate the habitat use and movement of sharks, and correlate those locations in time with environmental variables. Such studies provide valuable data for decision making in relation to marine environmental management.
In essence, acoustic tracking consists of equipping individual animals with acoustic transmitters and deploying an underwater array of acoustic receivers to detect when a particular individual is in the area. The logistical and technical challenges of tagging and tracking are formidable and the interpretation of the data can be anything but straightforward.
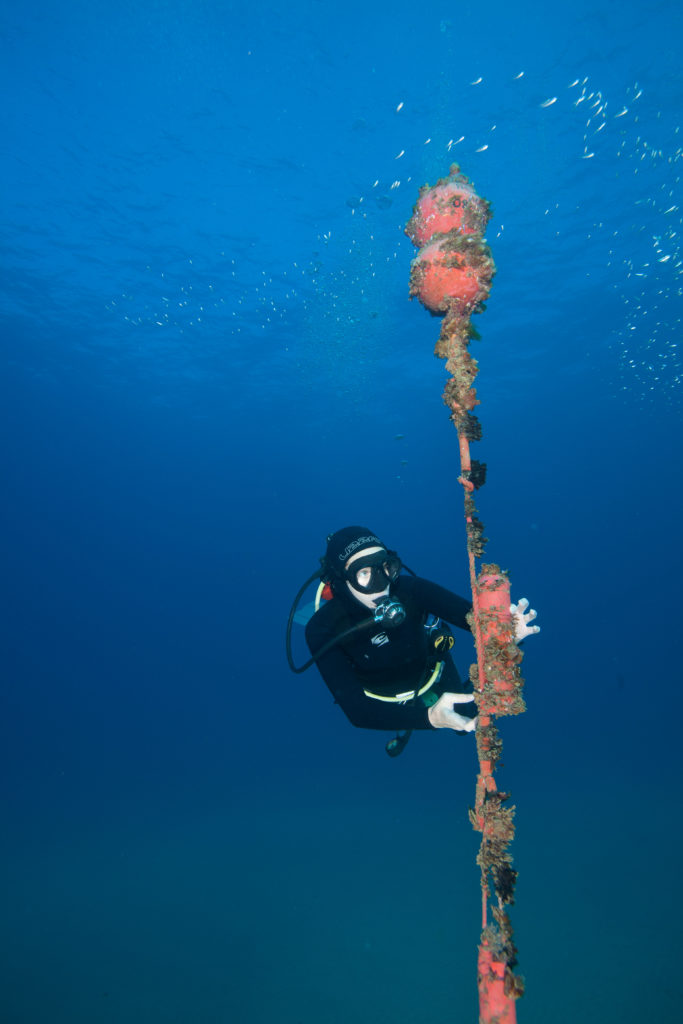
Determining the capability of each receiver in the array to detect a tag (its ‘range’) is a critical, but unfortunately also very difficult, aspect of the problem, as the detection range depends on a large number of environmental parameters, some of which vary with time. For example, high levels of ambient noise can mask the acoustic tracking signals, or density layers and other unfavourable water column properties can prevent the signals from travelling very far.
To help address this problem, MERIDIAN has partnered with the Ocean Tracking Network (OTN) on a project that seeks to analyze historical range testing data and model the efficiency of historical and proposed acoustic tracking arrays using machine-learning techniques. Using historical tracking data from OTN’s vast detection database, we will attempt to leverage the power of machine learning, e.g., neural networks, to uncover useful correlations between detection efficiency and various environmental variables.